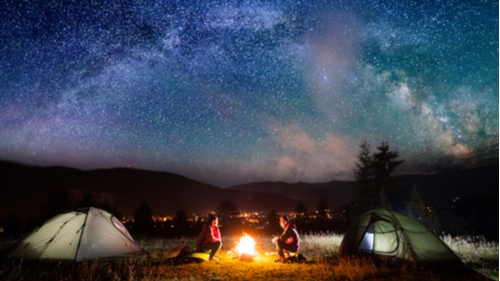
Peter Norvig is Director of Research for Google, and an expert in both artificial intelligence (AI) and online search. Prior to his work at Google, he worked at NASA, becoming the organization’s senior computer scientist.
He is a true AI Trailblazer, having literally written the textbook for AI. He authored Artificial Intelligence: A Modern Approach with Stuart Russell, which has been called “the most popular artificial intelligence textbook in the world.” He believes that an educational revolution, powered by technology, is pending and did more than most to foster it with his Stanford University class, “An Introduction to Artificial Intelligence,” with Sebastian Thrun. The class was made available to anyone in the world, and more than 160,000 students enrolled.
Norvig spoke in a fascinating Dreamforce Panel this year, entitled "How AI Is Transforming the Future Of Business", alongside Suchi Saria of Johns Hopkins and Richard Socher of Salesforce.
Over the last five years, artificial intelligence has become a huge topic of discussion in boardrooms, in the office, and in society as a whole. In our recent State of the Connected Customer report, we found that 65% of employees expect AI to have a major to moderate impact on their daily work life by 2020.
But AI is a topic given to extremes of emotion, and it’s sometimes challenging to determine what’s real and what’s hype. What’s your point of view on today’s AI state of play? What can companies use AI for today?
That’s a big question, and there are several ways to approach it. I think one way to break it down is by the uses of AI that are visible to the user, versus the uses that are not.
There are a lot of places where AI simply lets companies use their data better, and AI usage is largely invisible to the customer. Retail sites, for instance, can observe the products people are viewing, and use that data to begin to suggest other, more relevant products to them than was previously possible.
Machine learning helps companies do a better job of making those recommendations, but the customer doesn’t really notice that AI plays a role. They simply see their results getting incrementally better. This behind-the-scenes use of AI as an optimization tool for existing processes is an area where we’ve already made a lot of progress, and where the technology is pretty mature.
Then there are those use cases where AI is very visible indeed. One example is conversational agents like Google Home and Amazon’s Alexa — where a user simply says “hey, Alexa” or “hey, Google” and begins to interact by talking, rather than clicking buttons. From the user’s point of view, that’s very visibly different than what has happened before. With this type of visible AI, we’re just getting started. We’re not at quite the same level of maturity with the technology yet.
Then there are the areas where AI has enabled companies to provide a service with a 'magical difference' — a significant improvement in what was possible, with a very different user experience as a result.
Google Photos is a good example of AI providing that magical difference.Before, you would upload photos to a photo sharing site, and if you wanted to organize them, you went through the onerous task of putting them in folders, or labelling them with keywords.
Nowadays, you just dump your photos into Google Photos, and the system automatically labels them all for you. You can search for anything and it finds the right pictures without you having to do the tedious task of labelling all the photos. It frees you up to do the fun part: taking the pictures, sharing them with family and friends, and finding just the right picture when you want to go back to it.
That’s the way I look at how AI is having an impact right now. What’s available to a specific company depends on what its business is, how it interacts with its users, and what data it has.
I’d like to dive a little deeper into the ability of AI algorithms to process data and use it to make predictions and recommendations for a company or a customer.
Companies certainly seem to recognize the power of AI to improve their ability to use data for better predictions and recommendations, yet there is still a persistent skepticism on the part of the CEO to use data to make better business decisions.
According to a PwC survey of more than 2,000 business leaders, “most executives say their next big decision will rely mostly on human judgment, minds more than machines.” Just 35% of the executives surveyed say they rely mostly on internal data and analytics to make decisions. In large part, that seems to be because AI decision-making tends to happen in somewhat of a black box. Machine learning processes mean it’s very hard to see why an AI algorithm makes the recommendations it does.
What advice do you have for companies that are wrestling with that challenge right now?
That's a great point, and a lot depends on the particular use cases you have for AI.
I mentioned using AI to make recommendations of related products. It’s relatively simple in that case to do an A/B test to ascertain how well these AI-powered recommendations are doing. If the new AI algorithm does better than what came before, then it’s probably going to continue to do better in the future, and you can go with that.
It's a little bit harder to prepare for unforeseen problems, and to guard against big mistakes.
It's easy to say, “We increased click-through rate by 10% by using AI to power our recommendations.” It's hard to answer with certainty, “Is there going be a one-in-a-million mistake that gets us on the front page of The New York Times?” Those types of things are hard to predict because they just don't happen very often.
So you want to protect yourself against that sort of thing. But simply having more data can't do that on its own.
So what should companies do?
From my point of view, part of the answer is thinking about the user interface you have. Don’t make it look like your AI algorithm is saying anything with complete certainty. And ensure you have good customer support systems tied to any customer-facing AI, so you can quickly apologize when mistakes are made. Unfortunately, you’re never going to get to 100% accuracy.
Ultimately though, this is a trade-off between speed and safety. For instance, if you’re using AI to decide whether to show a social media post in a feed or not, you have a choice. You can ask yourself, “Do I want to be the very first? When I see an exciting-looking item, do I want to push that out to my customers right away?” Or do you want to be safer by saying, “I'm going to wait until I get confirmation from more sources, or more reliable sources.” There will always be this trade-off between potential positive returns and being slower-acting, but safer.
Companies looking to roll out AI algorithms for their own decision-making need to decide where they want to fall on that spectrum. Your comfort with risk will depend on what you’re using AI for. In the context of delivering content online, there are some instances where you can have humans in the loop, and others where it’s not feasible. If you're showing a small number of top items of the day, you can probably afford to have a human editor there who looks everything over, and gets rid of items that look bad. But if you're making recommendations for every single one of your million users, you can't afford to have a human in the loop.
This concludes part one of our interview with Peter Norvig. Part two, covering how to design AI for customer interactions, and the evolution of the workforce in the age of AI, is available here.
Peter Norvig was one of several AI luminaries to speak at Dreamforce 2017, alongside Vivienne Ming of Socos, Suchi Saria of Johns Hopkins University, Paul Daugherty of Accenture, and Richard Socher of Salesforce.