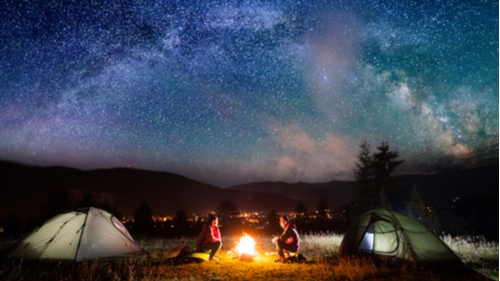
Suchi Saria is an assistant professor of computer science, health policy and statistics at Johns Hopkins University, where she directs the institution’s Machine Learning and Healthcare Lab.
Prior to joining Johns Hopkins, Saria completed her Ph.D. at Stanford with Dr. Daphne Koller. She was profiled in the MIT Technology Review as one of 35 innovators under 35, and is also a member of IEEE Artificial Intelligence's “10 to Watch” and Popular Science’s “Brilliant 10” lists.
A DARPA Young Faculty Award recipient, Saria found widespread acclaim as a result of her research into advancing new ways to use secondary sources of data like electronic health records to improve the way doctors diagnose and treat patients. For example, in sepsis, a life threatening reaction to infection, her work showed that it is possible to predict impending sepsis with significantly higher accuracy than existing screening tests.
Saria is a trailblazer in AI, and contributed to a fascinating Dreamforce Panel this year, entitled "How AI Is Transforming the Future Of Business", alongside Peter Norvig of Google and Richard Socher of Salesforce.
Your work on sepsis brought a 60% improvement over existing screening protocols. Businesses are already enormously excited about the potential for AI to drive a similar scale of improvements in their operations. Our own research suggests that 57% of IT leaders and 45% of service teams expect AI to have a “transformational or substantial” impact on their company by 2020 for instance.
But AI is not a cure-all. What sort of problems are AI and machine learning particularly well-suited to solving?
I think in the very near term, AI will be very useful to conduct routine tasks, where you have plenty of data, and where the risk of things going wrong is not high. Using off-the-shelf AI tools, one can make tremendous progress in a number of cases. For instance, tasks that have a lot of data available, and where being wrong is not going to be incredibly costly.
Common applications that people are already aware of are things like translation aides - where AI can listen for speech, and come up with a translation on the fly. Or for email, where AI can automatically determine who should be CC'd on any given message. It's a form of intelligent assistance that can be very useful.
We are also seeing progress in safety-critical domains like healthcare and autonomous driving. In this area, more carefully tuned algorithms are required. A big bottleneck that exists currently is the lack of trained individuals who can be hired to develop such software.
You’ve talked previously about doctors being concerned about machine learning making healthcare decisions. There is certainly skepticism on AI being given the capacity to make decisions in the boardroom as well.
In large part, that seems to be because AI decision-making tends to happen in somewhat of a black box. Machine learning processes mean it’s very hard to see why an AI algorithm makes the recommendations it does.
Do you have any advice for companies on how to navigate that skepticism and how to get decision-makers comfortable using AI?
I think that you will always need human decision makers on mission-critical decisions, or in instances where there isn’t a clear protocol for decision-making.
But in other areas, it depends on what you’re asking an AI algorithm to do. If you have, for instance, millions of samples to analyze, AI can help in discarding irrelevant samples and focusing on those you should focus your attention on. In that case, the AI isn’t actually making a decision, it’s simply triaging to a smaller set of samples.
A good example is credit card fraud detection. It's very hard to hire enough people to go through all those credit card statements. But it's easy if there's another computer that is looking for candidates. Then a human can look at only the suspicious candidates that the computer identifies. The computer gets rids of 80% of the data, leaving you to focus your attention on the 20%.
What you're saying is, as it stands, AI is great for extending the bandwidth of a human agent rather than replacing them altogether?
Absolutely. And specifically extending bandwidth in areas where there is a low cost of error.
You're giving employees the ability to do things they would rather not do themselves, or they would get pretty fed up doing. If an AI could help me with triage, I could get on with more high-value tasks. Effectively, we’re partitioning a job into a series of tasks, where the human is taking on more of high-end cognitive task and leaving the more routinized task to AI.
There are plenty of people who are concerned about the impact of AI on the workforce of tomorrow. In fact, 65% of employees expect AI is going to have a major or moderate impact on their daily work life by 2020.
You appear to agree with Vivienne Ming and Peter Norvig, that it’s better to think of AI as something that will complement human workers, rather than simply replace them wholesale.
Is that right?
This idea of a technology that could impact jobs is something we’ve seen before in many fields. In the past, we’ve tended to find a way to use that technology to become more productive. We do more. We consume more.
A classic example is farming. A hundred years ago, 26% of the population used to be involved in farming. Now, only 1% of the population is involved. Yet, our product is higher than what it used to be 100 years ago. So we eat more. We eat nicer things. And we have far more individuals in the service industry, using the benefits of that progress. Nutrition has expanded considerably as a field, for instance.
Hopefully, AI’s impact will be in the same vein, and this new revolution will be about allowing people to do more. AI has the capacity to free people up from basic rote tasks, such that they can become more productive, and do things faster.
A radiologist can analyze many more images, and they can spend more time on some of the harder cases. There are some really nice examples in cardiology, where you look at electronic traces and try to figure out if there's arrhythmia. Going through those traces is very hard. But with an AI system that can analyze and identify irregular heart rhythms, then the cardiologist can focus on those cases, and really spend time understanding them more deeply.
That general idea is true almost anywhere.
Suchi Saria was one of several AI luminaries to speak at Dreamforce 2017, alongside Vivienne Ming of Socos, Paul Daugherty of Accenture, and Richard Socher of Salesforce.