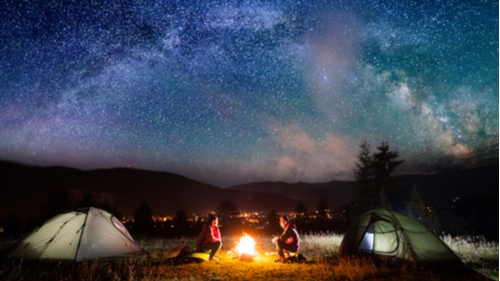
In 2013, Casey Bennet and Kris Hauser, two researchers from the School of Informatics and Computing at Indiana University, created a simulation to model how patient outcomes and healthcare costs could be impacted by machine learning. An AI algorithm detected patterns and made diagnoses based on a patient’s history and data from millions of other patients, and recommended appropriate treatment plans to a human doctor based on the latest medical literature and predictive models.
Those AI-simulated plans improved patient outcomes by 50%, and did so while reducing healthcare costs by 50%, compared to current treatment regimes.
In 2016, as the world’s media excitedly reported, Google’s computer program AlphaGo beat reigning human world champion Lee Sedol at Go — considered to be the most challenging strategy game on the planet. The research team behind AlphaGo is now developing new algorithms to solve real-world business problems, and has entered into a partnership with the U.K.’s National Health Service to increase the accuracy of analyses and build better systems to alert clinicians to important patient data at the right time.
In 2017, AI-powered hedge funds easily beat human-run alternatives, achieving annual returns of 8.44% over the last six years. Another study found that AI algorithms were considerably more successful than human pathologists in diagnosing breast cancers.
There’s a growing body of evidence to suggest that AI has a powerful role to play in making real-world decisions. It’s unsurprising to learn that many of the most innovative organizations in the world — such as Facebook, Google, and Amazon — rely on AI algorithms as part of their decision-making process.
According to Amazon CEO Jeff Bezos, “Basically, there’s no institution in the world that cannot be improved with machine learning.”
The benefits of giving AI a role to play in business decision-making are many:
Faster decision-making: In a world where the pace of business has accelerated and shows no sign of slowing down, the ability to speed up the decision-making process is crucial. For example, with AI-powered pricing, oil companies can dynamically change the price of gas according to demand, improving their margins by around 5%. Similarly, retailers, travel sites, and other services routinely use dynamic pricing to optimize their margins.
Better handling of multiple inputs: Machines are far better than humans at handling many different factors at once when making complex decisions, can process much more data at once, and use probability to suggest or implement the best possible decision.
Less decision fatigue: Multiple psychology studies show that as individuals are forced to make multiple decisions over a short period, the quality of those decisions deteriorates over time. It’s why supermarkets place candy and snacks at cash registers. Exhausted by all the decisions made during a shopping trip, shoppers find it much harder to resist the lure of a sugar rush at the point of sale. Algorithms have no such weaknesses, and can help executives avoid making poor decisions borne of exhaustion.
More original thinking and nonintuitive predictions: AI helps executives spot patterns that may not be readily apparent to human analysis. For instance, a major drugstore discovered through AI that people who bought beer also tended to buy diapers at the same time. This kind of unique insight can have immediate impact on a business.
There is now a significant collection of research from over the last 70 years that, according to Deloitte, has established that even simple predictive models outperform human experts’ ability to make predictions and forecasts.
Yet, many CEOs are reluctant to hand over decision-making duties to predictive models and algorithms. Michael Schrage, a research fellow at MIT, described one multibillion-dollar company where it was shown that by all measures and simulations handing AI the ability to make decisions on procurement would save hundreds of millions of dollars. But the company CEO was nevertheless unwilling to take the plunge.
That CEO is not alone. According to a PwC survey of more than 2,000 business leaders, “Most executives say their next big decision will rely mostly on human judgment, minds more than machines.” Just 35% of the executives surveyed say they rely mostly on internal data and analytics to make strategic decisions.
While it would be remiss to suggest that CEOs should rely exclusively on AI to make their next strategic decision, it seems business leaders are missing an opportunity to fully exploit AI to assist their decision-making.
Their reluctance is borne of three main factors:
Accountability: Many algorithms suffer from the black box problem, in which there is no explanation for how that algorithm derived its answers. C-level leaders are hesitant to put blind trust in those algorithms to make critical decisions for them, and require more accountability and transparency from AI systems than many can currently offer.
Bias: We worry about human bias, but AIs are the product of humans and are also susceptible to bias. Research recently published in Science on “word embedding,” a machine learning tool that helps computers make sense of language by looking at a word’s association with other words, found that words like “woman” were closely associated with the arts, while “man” was closer to engineering professions. Given AIs are “trained” on datasets that may contain bias, and by computer scientists who may be biased, there is a risk that algorithms may produce overly biased results.
Pride: CEOs have become leaders by relying on their own judgment and finding it to be sound. Some may be less inclined to follow the advice of a computer, even an intelligent one.
Yet, as cognitive scientists Richard Nisbett and Lee Ross say in their book Human Inference, “Human judges are not merely worse than optimal regression equations; they are worse than almost any regression equation.” So how can business leaders most effectively leverage the power of AI to make better decisions?
PwC uses the metaphor of a self-driving car to describe how companies can begin to leverage AI for better decision-making. In its example, the autonomous car is used to represent the business, while the passengers are the business’s executives.
Those executives set the destination, and can at any time override the car to make changes they feel are necessary. But once in motion, the car is learning far more from its environment than a human driver ever could, and using many different external data sources to plot the most efficient route to a destination.
For any company looking to leverage AI to drive better decision-making, this partnership metaphor is useful. AI can process information at a scale and scope beyond human capacity, and it is thus a considerable boon to companies looking to improve the speed and accuracy of their decisions. But humans must always be involved in that decision-making process, defining the questions to be asked, and having a final say on the best answer for their business. While machines are superior at handling large volumes of data at speed, humans are still stronger at analyzing a decision in the context of the real world. They are the stronger partner at exercising judgment based on emotion, empathy, and social norms.
We know, for instance, that AI is faster than humans at dynamic pricing. One need only consider Orbitz, who got into hot water for charging Mac users more than PC users, or Uber, whose prices doubled during terrorist attacks in London in June, to see that humans should have oversight on whether instant dynamic pricing is a good idea in the first place.
As Anand Rao, an innovation lead in PwC’s data and analytics practice, said:
“First, people teach machines what to do, but then the machine advises people what they should do. Because the machine is teaching — telling people what to do — the people get smarter and can tell the machine additional things it should do. Each one is helping and augmenting the other.”
This feedback loop makes the most of people and machines, and is key to keeping any CEO in the driver’s seat.